Principal Investigator
Xavier Pennec
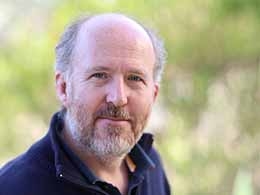
Xavier is a research director at Inria. His interests lie at the intersection of differential geometry, statistics and computer science with applications to Medical Imaging.
xavier.pennec[at]inria.fr
Starting Position
Guillaume Olikier
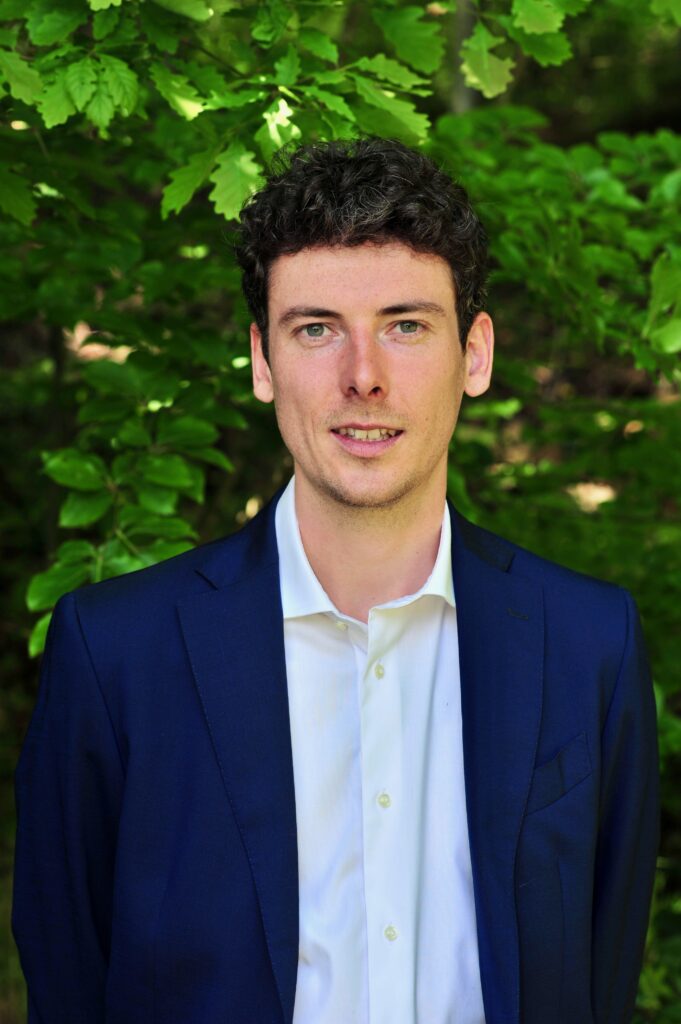
My main research area is numerical optimization, with a particular interest in the design and analysis of optimization algorithms on stratified sets based on tools from variational analysis. Examples of such sets include the set of sparse vectors, its intersection with the nonnegative orthant, the determinantal variety (i.e., matrices of bounded rank), its intersection with the cone of positive-semidefinite matrices, and tree sets. These sets appear in numerous applications such as compressed sensing, matrix completion, and statistics. Ongoing work aims at better understanding the behavior of optimization algorithms on stratified sets by identifying relevant properties of these sets.
guillaume.olikier [at] inria.fr
James Benn
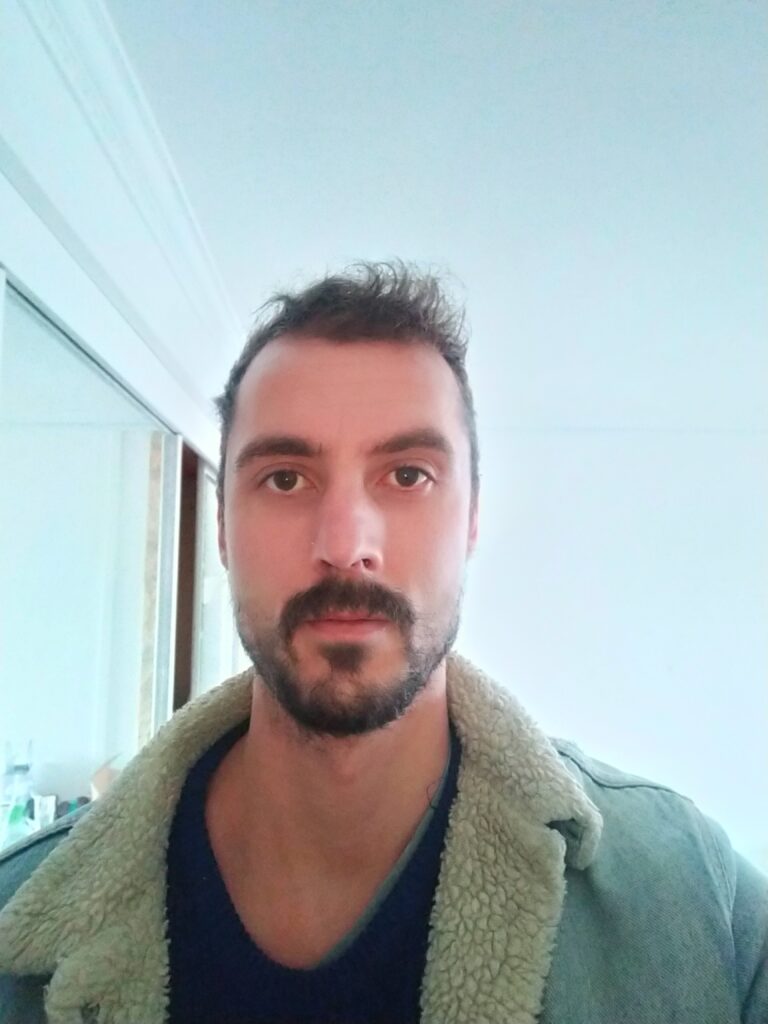
I am interested in applications of geometry and analysis to problems in shape representation and their statistical analyses. Current work in this area includes the modelling of probability distributions on manifolds along with surprising applications in hydrodynamics. In collaboration with Anna Calissano and Xavier Pennec, we are exploring alternative graph representations which are suitable for statistically analyzing populations of graphs and developing dimensionality reduction techniques. I am also interested in Lagrangian and Eulerian stability on diffeomorphism groups and their applications to uncertainty quantification of diffeomorphometry frameworks such as LDDMM.
james.benn[at]inria.fr
PhD Students
Olivier Bisson
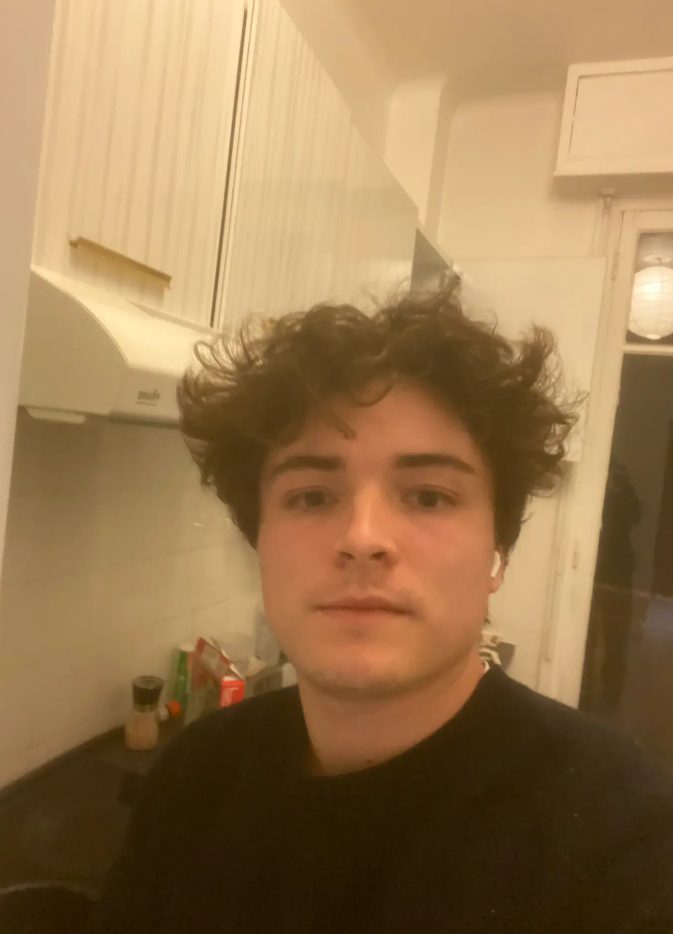
I am interested in studying the various geometries of the open elliptope of correlation matrices. In particular, I study how to develop and implement Riemannian metrics over correlation matrices and then how to perform statistics within such spaces. This work includes studying the stratification of the correlation matrices, from various geometrical perspectives. I also investigate how well these geometric structures impact the application problems in neuroimaging.
olivier.bisson [at] inria.fr
Tom Szwagier
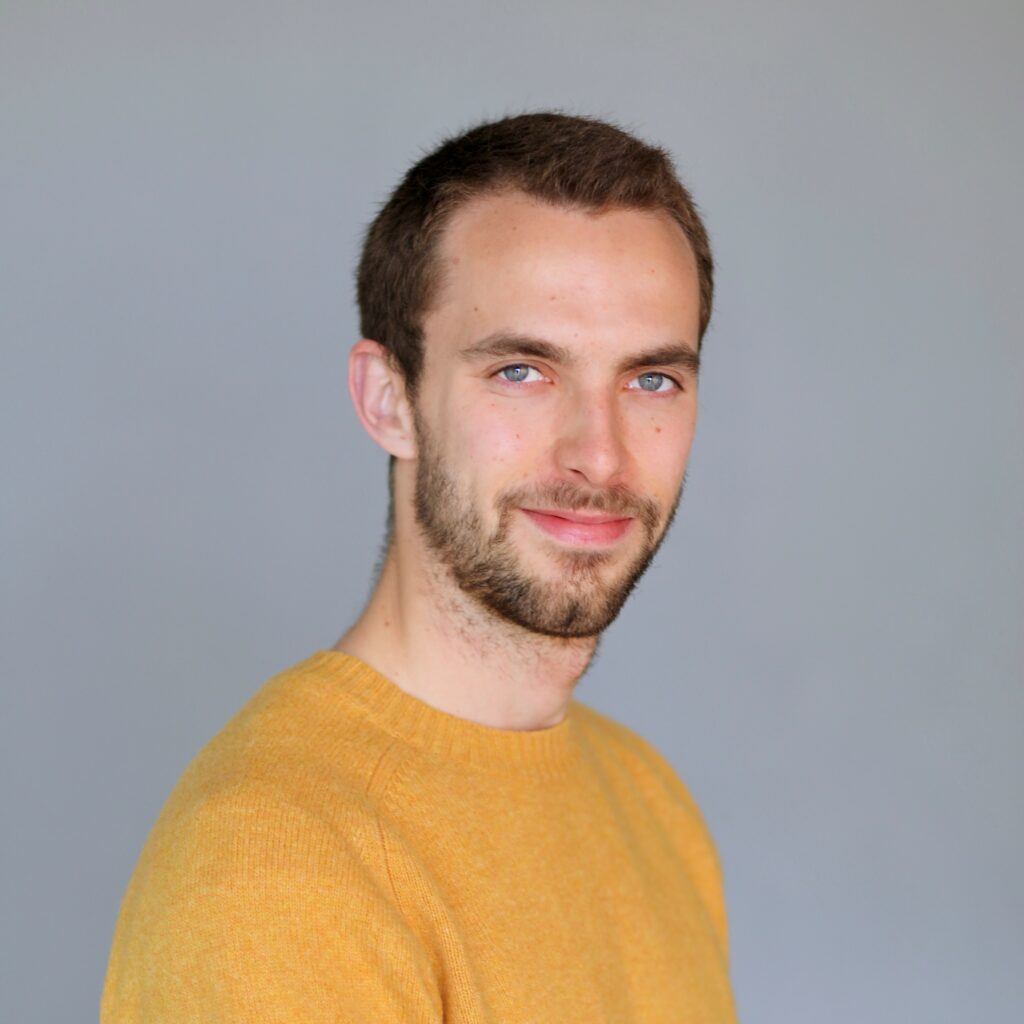
I study dimension reduction methods from a geometric point of view. I am particularly interested in flag manifolds and Grassmannians which, when provided with the right tools for Riemannian optimization, enrich the classical methods like Principal Component Analysis. I am also interested in Barycentric Subspace Analysis as an hybrid method for dimension reduction and clustering.
tom.szwagier [at] inria.fr
Alumni
Morten Pedersen
I am constructing new methods for dimension reduction using differential geometry, especially sub-Riemannian geometry and stochastic processes on manifolds. The input for the methods can be data in Euclidean space (vectors in \R^d), from which we want to estimate a non-linear submanifold approximating the data. It can also be data residing on an a priori known Riemannian manifold, from which we estimate an approximating submanifold. One example of this is a method for doing dimension reduction of observations residing in shape spaces, and which are assumed to evolve randomly following the structure of a phylogenetic tree. I have constructed a method (‘tangent phylogenetic PCA’) which generalizes PCA to this setting of shape-valued observations while taking into account the non-trivial correlation induced by the tree structure.
Email: morten.pedersen [at] inria.fr
Elodie Maignant
I joined the project in October 2020 for a PhD focused on geometric manifold learning. I am working on designing intrinsic methods for the analysis of manifold-valued data with a particular interest for shape data and graph data. I leverage Riemannian models and explore non-metric structures such as barycentric subspaces. Additionally, I look for approximations by constant curvature spaces. I am also supervised by Alain Trouvé.
Email: elodie.maignant [at] inria.fr
Anna Calissano
My research focuses on the definition of suitable geometrical embeddings and statistical methods for the analysis of set of graphs. Such graphs are often unlabelled, requiring an alignement between the nodes across the population. Such unlabelled graphs are often described in quotient spaces, requiring tools beyond manifold statistics. My main applications are brain connectivity graphs, public transport system networks, and shape graphs.
Email: anna.calissano [at] inria.fr
Luís F. Pereira
I’ve joined the project in April 2022 and I’m the maintainer of `geomstats`, our open-source Python package for computations and statistics on manifolds with geometric structures. I’m deeply interested in scientific computing. My main focus is to ensure we implement the right abstractions (both from a mathematical and computational point of view) with clean, readable, maintainable and correct code.
Email: luis.gomes-pereira [at] inria.fr
Dimbihery Rabenoro
Dimby joined the project in May 2020 to work on the uncertainty of the estimation of the mean and higher dimensional sub-spaces in extensions of PCA to manifolds. Dimby holds a PhD in probability (large deviations theory) from University Paris 6.
Email: dimbihery.rabenoro [at] inria.fr
Andreas Abildtrup Hansen
Andreas visited G-Stats from September to November 2022. He is working on Equivariant and Invariant modelling for set of graphs (POP Nets).
Supervisor: Prof. Aasa Feragen, Anna Calissano
Yann Thanwerdas
Yann joined the project in september 2018 for his Master’s thesis and started his PhD in january 2020. His focus is on Symmetric Positive-Definite matrices and applications to Neuroimaging.
Email: yann.thanwerdas [at] inria.fr
Nicolas Guigui
Nicolas joined the project in october 2018 for a PhD focused on computational methods for statistics on manifolds and symmetric spaces.
Email: nicolas.guigui [at] inria.fr
Raphael Sivera
Raphael was part of the project between January and June 2019 before finishing his PhD. He developed multivariate statistical methods to assess a treatment effect on the longitudinal deformations of the brain, relying on the Stationary Velocity Field framework and parallel transport.
Bastien Manach-Perennou
Bastien did his Master’s thesis in the team between September 2018 and June 2019. He worked on transitivity of rigid-body registration procedures.